The Risks And Opportunities Across The AI Value Chain

Reviewed by Michael Paige, Bailey Pemberton
Quote of the Week:
"AI will probably most likely lead to the end of the world, but in the meantime, there'll be great companies." - Sam Altman
Following on from Part 1 last week where we covered the arguments for and against AI being in a bubble , we are taking a look at the entire AI value chain to see where investible opportunities might pop up, and what the risks are.
If Generative AI is akin to the most important tech innovations of the past ( think: the PC, the internet, and smartphones ), then many of the best investments might not even exist yet, or they are likely to be small startups you haven't heard of.
So you don’t need to rush out and buy today’s market leaders, but it's worth knowing what type of companies to look out for.
What Happened in Markets this Week?
Here’s a quick summary of what’s been going on:
-
🇯🇵 Nikkei 225 Index Makes New Record High for First Time in 34 Years ( FT )
- Our take: Japan is benefiting from worsening relations between China and the West, and the AI boom. The likes of Tokyo Electron and Advantest (a supplier to NVDA and others) have seen their share prices double in USD terms over the last year. Remember when we said last week "Look for where the Magnificent Seven’s cash is flowing" ? These two companies have the 2nd and 3rd largest weightings in the Nikkei 225, which is a price weighted index similar to the Dow.
-
📺 Walmart is Buying Smart TV Maker Vizio to Expand Ad Business ( Fast Company )
- Our take: This could turn out to be a great move for Walmart’s margins, because advertising is a great business - while retailing is a very difficult, low-margin business. Walmart earned $15.5 billion in net income in the last 12 months, while Amazon’s ad business alone generated $49 billion in revenue, with relatively low costs (potentially making as much profit as AWS if you believe this narrative on Amazon ).
-
💳 Capital One’s $35B bid to buy Discover would create America’s largest credit-card lender ( Yahoo )
- Our take: Visa and Mastercard might have some competition coming. By merging Capital One’s 100M customers in the US, UK and Canada, with Discover’s credit card network used by 350M+ cardholders, the combined company could put up a fight against the incumbents in the payment processing business. Seems like Capital One is betting that credit card use will continue to grow, and they’d be benefitting from the higher interest rates and the big increase in credit card debt we’re seeing ($1.1T in the US).
And some of the key economic data released recently:
-
🇨🇦 Canada’s inflation rate fell to 2.9% from 3.4%.
- This was even lower than the 3.2% expected by economists and raised hopes for a rate cut in April. Shelter price inflation was still very high at 6.2% as rental rates continued to rise. Stripping out this component puts the inflation rate at just 1.5%.
-
🇯🇵 Japan’s trade deficit shrank to ¥1.76 trillion yen ($12bn USD) after exports rose 12% in January.
- Imports for things like oil, natural gas and iron ore fell 9.6% which also contributed to the lower deficit, which is down nearly 50% from a year ago.
-
🇺🇲 The minutes from the last FOMC meeting held few surprises and indicated that the committee is wary of cutting rates too soon.
- Members were optimistic that their decisions had succeeded in bringing inflation down, but “most participants noted the risks of moving too quickly to ease the stance of policy.”
💰⛓The Generative AI Value Chain
Last week we contemplated the likelihood that AI stocks are now in bubble territory. Expectations for the leading AI stocks are certainly very high, and that always raises the risk that returns turn out to be disappointing.
If you are building your exposure to AI, one way to manage this risk is to consider companies lower down the value chain, where expectations aren’t as high.
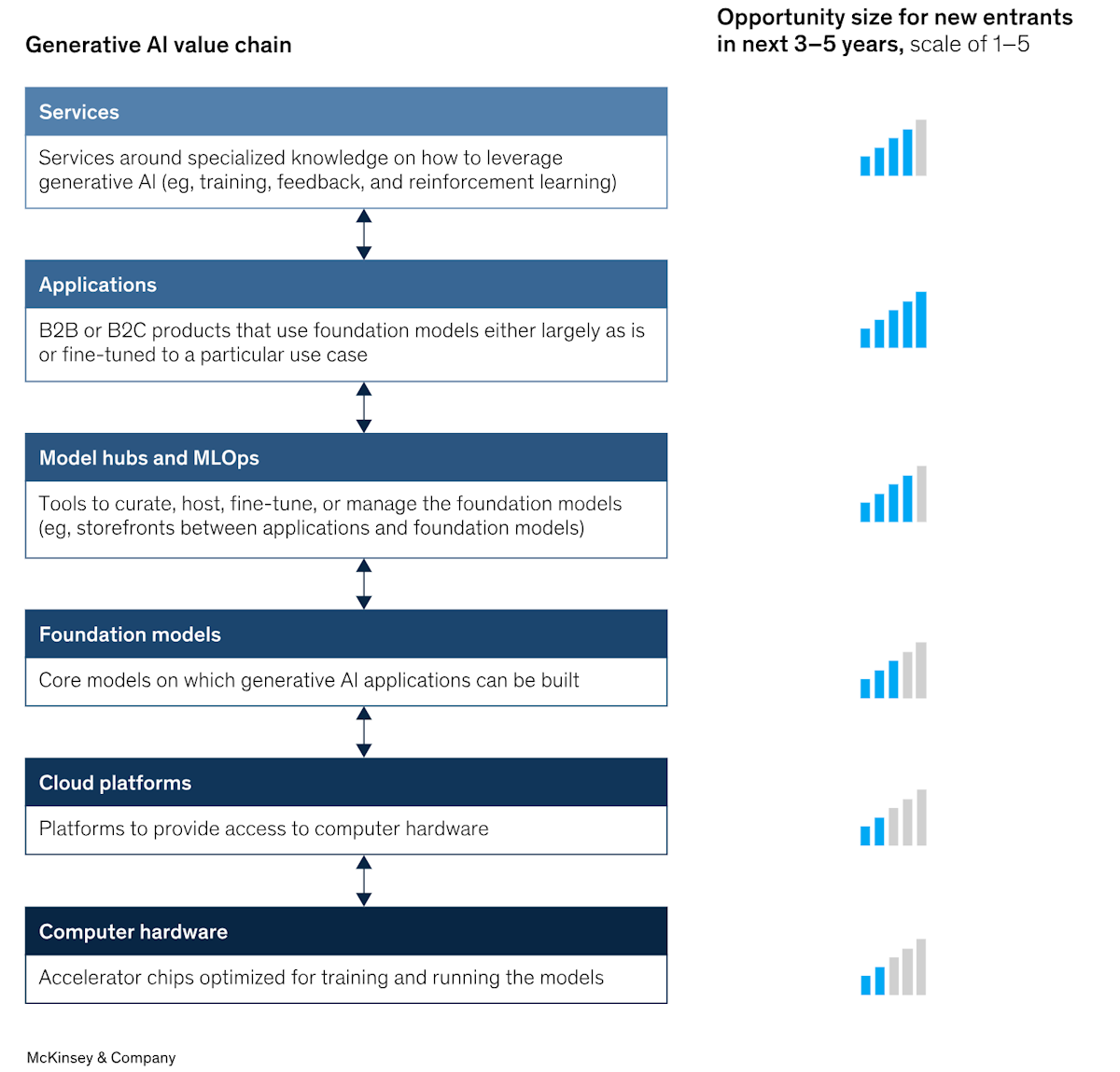
McKinsey & Company has identified six levels to the emerging generative AI value chain .
✨ While most of the attention is now on the bottom three levels, McKinsey believes more value will ultimately accrue to the top three levels.
This may not seem obvious now, but previous innovations have followed a similar pattern. The early beneficiaries of the internet were companies like Cisco - but it was new companies like Amazon and Google that created value over time.
Also, it’s worth noting that the three lower levels are incredibly capital intensive, which is one of the reasons they are dominated by large companies with deep pockets. As the industry evolves, hopefully there will be room for more companies to participate.
Let’s look at some of the types of opportunities and potential risks within those six levels.
💾 Computer Hardware
LLMs (large language models) use massive amounts of processing power. At the center of this are the GPUs, but data centers also require all sorts of other hardware and networking equipment.
🔎 The Opportunities
Nvidia is the leading supplier of GPUs so it’s no surprise that it’s front and center of the AI revolution. AMD is the underdog here, and TSMC is the company that actually manufactures most of the GPUs.
The other companies with exposure to this space are those that sell servers, networking equipment and other hardware used to run AI models. Examples include Super Micro Computer , Arista Networks , A10 Networks and Cisco .
Other companies within the semiconductor ecosystem are ASML , Cadence and Synopsys .
⚠️ The Risks
There’s little doubt that companies like Nvidia will sell a lot of GPUs in the next few years. The risk is that revenue or margins don’t live up to what the share price is expecting.
For other companies that supply hardware to datacenters, there’s a risk that they don’t have enough unique IP to protect their margins. That’s not to say building servers and networking equipment is easy, but it’s not quite in the same league as designing or manufacturing GPUs.
☁️ Cloud Platforms
The big tech companies that offer compute power and storage have managed to benefit from most of the new innovations of the last decade, and once again they are front and center of the AI revolution.
🔎 The Opportunities
The largest cloud providers have countless advantages when it comes to capitalizing on AI. They have the capital and resources to develop models, they provide compute power and other services, and they already have millions of customers to sell new AI services to. Another way they can benefit from AI is by cutting their own costs.
There are also companies that focus specifically on building out or owning the physical infrastructure or properties. Some of these include REITs like Equinix , Digital Realty Trust and American Tower Corporation . Another example is private equity giant Blackstone which is investing $25 billion in a series of AI data centers.
⚠️ The Risks
The big tech companies are fortunate in that they are very well diversified. That said, they are unlikely to be immune if AI falls out of favor.
For other companies, including the REITs there’s always a risk that they trade on hype rather than fundamentals - so make sure you understand the valuation and how realistic it is.
🏗️ Foundation Models
The foundation generative AI models are massive pretrained models like GPT, Gemini and Llama. These models are trained to use text as an input, and then output content including text, audio and video. Applications like ChatGPT use these models as an engine to create specific types of content.
🔎 The Opportunities
Building and training LLMs requires expertise, capital to access the compute power required to train a model, and data to train them on. So once again this is mostly the domain of very large companies - as well as startups like OpenAI and Anthropic that manage to partner with them.
It’s no surprise to see Microsoft , Alphabet and Meta dominating here. But there are a few dark horses like Apple and even X that could enter the fray.
In time the requirements may become more affordable as chips become more powerful, so smaller companies may be able to compete.
⚠️ The Risks
As we mentioned last week, LLMs face the risk that they struggle to maintain a competitive advantage. This is particularly true with the emergence of open source models like Meta’s Llama.
🧰 Model Hubs and MLOps
Model hubs refer to the platforms or tools used to host and manage models - or in many cases to access models owned by cloud platforms. MLOps, or Machine Learning Operations, refers to the process of developing, deploying and managing machine learning applications.
✨ This part of the value chain is easy to overlook, but it’s incredibly important. Developing AI applications is expensive and requires meticulous planning, testing and management. The fact that the technology is evolving so quickly makes it even more critical.
🔎 The Opportunities
All the cloud providers provide some level of MLOps tools and services. But there are lots of other companies that provide similar services.
These include legacy IT companies like IBM , Kyndrl (which was spun off from IBM) and HPE, as well as AI and data platforms like Palantir, C3.ai and Snowflake . It’s likely that data platforms will continue to offer more AI solutions over time.
There is also a growing list of startups that provide MLOps services. These include Databricks (which is rumored to be moving towards an IPO) and Hugging Face.
⚠️ The Risks
If you are investing in companies in this space, make sure you understand the business model, and how much of their revenue is recurring. Consulting businesses have low capital requirements, which is great, but they don’t always have the predictability of a subscription business.
📲 Applications
If AI follows a similar pattern to previous tech innovations, the companies that develop new apps will create the most value for customers and shareholders.
Large language models can seem like a blunt instrument at times. They can create content that looks great, but can be full of inaccuracies. That’s fine when it comes to writing a story, or creating music or video content. But when facts matter they need to be fine-tuned and often new data needs to be introduced.
✨ If foundation models are the engine, the applications that they power are like the rest of the vehicle including the transmission, the brakes and the tyres. These can vary depending on the intended use: is the vehicle a sports car or a pickup truck?
Gen AI applications can solve a variety of problems for consumers and for different industries. To do this they need an interface, and they need to be fine-tuned. They also need access to relevant information.
Let’s consider a bank’s customer services chatbot. It’s all very well that it can have a conversation with a customer, but it also needs to be able to access the customer’s account details and previous interactions. It also needs to know what information it can’t share. To be really effective, a chatbot needs to be fine-tuned and trained on the right data and the prompts need to be engineered to produce the right output.
🔎 The Opportunities
Anyone can create an app powered by AI. but some companies have advantages that individuals and small startups don’t.
- Resources like capital and expertise are a good start.
- A large customer base or audience is even better, and
- Access to lots of proprietary data to train and fine tune models really creates an unfair advantage.
Meta , Alphabet , Amazon and Microsoft tick all the boxes once again. Other companies that tick all these boxes are Salesforce, PayPal, Shopify, Tencent, Alibaba etc.
For most of these companies, the AI opportunity is at least partially baked into the price. One company where the AI opportunity might not be in the price is Apple . Analysts are currently forecasting earnings and revenue growth of to 5%. That could change if or when Apple starts releasing AI products or services.
Some analysts are already calling Apple the dark horse of the AI race . The company is working on its own LLM, and Siri is rumored to be getting a generative AI makeover. More importantly, Apple already has 2 billion devices in customers hands and the App Store, so it can profit from third party apps. Right now, there’s little clarity on all of this - but it’s one to watch.
⚠️ The Risks
Barriers to entry are fairly low for AI app development, so it’s likely to become very competitive. If the AI rally ends with a speculative small cap frenzy (like it did with dotcom stocks, EV stocks, cannabis stocks etc) this is likely to be the part of the market where that happens.
✨ Make sure any companies you invest in really do have competitive advantages and a sustainable growth path.
🛠️ Services
At the top of McKinsey’s Gen AI value chain are the companies that provide services to companies developing and deploying gen AI solutions. Many of these companies are the same as the ones that provide MLOps services.
🔎 The Opportunities
Analysts at Mizuho Securities recently highlighted IT services companies as an alternative way to invest in AI. They pointed out that these companies have already been advising corporations on digital transformation, so they already have the right relationships.
IBM , Kyndrl and HPE all operate in this space. Other companies they mentioned are Accenture , Globant and EPAM Systems . These types of companies are usually classified under IT Consulting, though some may fall within other industries.
The image below from the Simply Wall St Markets Page reflects the PE ratios and forecast growth rates for the IT Consulting industry, tech sector and the broader market.
This tool is a great way to compare expectations (in the form of PE ratios) and growth forecasts across industries and sectors.
⚠️ The Risks
While these companies are exposed to AI, they aren’t movers or shakers, so they’ll always be on the periphery. This is great in that if the AI bubble bursts, you’re less likely to see a catastrophic downturn in the share price of these companies.
But by the same token, you aren’t likely to find an elusive 10-bagger here, as the value they add is incremental and the work they do is supportive, rather than disruptive.
💡 The Insight: Don’t Forget The Corporate AI Adopters
When we look at the application layer of the value chain, the first companies that come to mind are those providing apps and services to consumers and companies. The companies using those apps, and in many cases creating their own apps, can also benefit in very tangible ways.
Here are a just few examples:
- 🗒️ Admin: Greater efficiency and lower costs.
- 🛠️ Product development: Faster prototyping and development.
- 📺 Marketing: Personalized content creation and insights.
- 💁Customer service: Greater engagement at a lower cost with chatbots.
With the right tools, companies can increase revenue and reduce costs. Companies with lots of admin related costs have a lot to gain by reducing costs alone. Others that stand out are companies that generate a lot of data, which they can leverage to provide insights.
✨ This could apply to most industries, but banks, insurers and healthcare providers stand out. Companies outside the technology sector that benefit from AI would also be less exposed to the fallout from an AI bubble bursting.
Stay tuned next week - we will be going over some use cases and real world examples of generative AI in action, as well as its limitations.
Key Events During the Next Week
This week, there’s plenty of US data that will reflect the strength of the economy ahead of the Fed’s next meeting.
Monday
- 🇺🇸 US New home sales are expected to rise just 0.9% month-on-month, after previously jumping 8%.
Tuesday
- 🇯🇵 Japan’s inflation rate is expected to fall to 2.4% from 2.6%.
- 🇺🇸 US Durable good orders are expected to be 4% lower.
Wednesday
- 🇺🇸 The second estimate for US GDP for Q4 2023 is expected at 3.3%, down from the 4.9% result recorded for the third quarter.
Thursday
- 🇪🇺 In Europe, there’s inflation data due in France (2.9% expected vs. 3.1%) and Germany (2.8% expected vs 2.9%)
- 🇨🇦 Canada GDP growth rate will be published. It’s expected at -0.2% compared to the prior -0.3%.
- 🇺🇲US personal income, spending and the PCE price index will be published. Income and prices are expected to be flat MoM, while spending is expected to slow.
Friday
- 🇺🇸 The University of Michigan Consumer Sentiment Index is expected to come in at 79.6, a slight improvement from the prior reading of 79.
It’s one of the last big weeks for earnings season, with cloud software stocks in focus:
Have feedback on this article? Concerned about the content? Get in touch with us directly. Alternatively, email editorial-team@simplywallst.com
Simply Wall St analyst Richard Bowman and Simply Wall St have no position in any of the companies mentioned. This article is general in nature. We provide commentary based on historical data and analyst forecasts only using an unbiased methodology and our articles are not intended to be financial advice. It does not constitute a recommendation to buy or sell any stock and does not take account of your objectives, or your financial situation. We aim to bring you long-term focused analysis driven by fundamental data. Note that our analysis may not factor in the latest price-sensitive company announcements or qualitative material.

Richard Bowman
Richard is an analyst, writer and investor based in Cape Town, South Africa. He has written for several online investment publications and continues to do so. Richard is fascinated by economics, financial markets and behavioral finance. He is also passionate about tools and content that make investing accessible to everyone.